Solving optimal stopping problems with statistical algorithms
Enough Is Enough
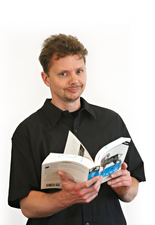
When is it statistically favorable to conclude, say, the search for a suitable employee with a chosen one? Solid algorithms point the way to success.
The saying goes that you should quit when you're ahead. Making a decision on when to conclude a selection process is often not that easy in real life. In mathematical statistics, a puzzle known as the "secretary problem" [1] sums this up nicely. A number of candidates are applying for a secretary position at a company. In the selection process, the employer must decide after each interview, whether to accept the candidate or reject them and hope for a more suitable applicant later on. The decision is final, the employer is not allowed to invite back any rejected applicants.
An employer who is thinking logically should carefully check the first few candidates and not simply take the first one. Later, when the line starts to come to an end, the employer will possibly take on a more-or-less suitable candidate for fear of being left with only unsuitable candidates, and – not having any other options – grudgingly just bite the bullet, and grab whoever is left.
Ideal: 37 Percent
How should the employer proceed to mathematically ensure the best possible odds of finding an above-average candidate? How long should the try-before-buy phase last, in which the employer explores the unknown capabilities of candidates before immediately snapping up the one that is better than all the previous ones and hopefully scores favorably against future candidates further down the line? Mathematicians have been tackling stop problems [2] for thousands of years.
As the recently published book Algorithms to Live By [3] entertainingly explains, the answer is 37 percent, or more precisely 1/e * 100%
. Given 100 candidates, an employer is well advised to check out the first 37 without any intention to employ anyone, just to gain some idea of the talents available on the labor market. As of candidate number 38, smart bosses then immediately sign a contract if one candidate is obviously better than all the previous ones.
Love-Crazed Stargazers
This task is also known as the wedding problem. The story of the famous star gazer Johannes Kepler, who was desperately looking for a new wife after the death of his first wife in 1611, is legendary. With meticulous mathematical skills, he launched himself into a lengthy process of research, looking at no less than 11 candidates. Completely exhausted and remorseful, he then returned to candidate number five, proposed to her, and to his great joy luckily received a glowing "Yes!" as an answer to his question.
Simulating Reality
To work your way through the secretary problem with a Perl program, the script needs to make some assumptions about the distribution of the supply in the labor market. The controversial "20-70-10" rule of American tycoon Jack Welch [4] dictates that the 20 percent top performers in a company return exceed expectations, 70 percent work quite well, and 10 percent are unproductive. For the experiment, we let the labor market also follow this scheme; the available talents thus roughly lie on the bell curve of a Gaussian normal distribution (Figure 1).
Because the candidates arrive in random order, the script needs a random generator that outputs a normally distributed pool of talents. The random_normal
function of the Math::Random
CPAN module already has such a generator; in Listing 1 [5], it uses the parameters 100_000, 10, 2
to return 100,000 random values that fluctuate around the mean value of 10 with a standard deviation of 2.
Listing 1
histo-normal
To check whether the distribution also roughly meets the requirements visually, Listing 1 uses the get_histogram()
function from the Statistics::Histogram CPAN module to print a histogram of distributed values as ASCII art on the command line. The bell shape is indeed visible (Figure 2), and although the maximum of the random data is at around 10, the frequency drops off towards both sides, and there are hardly any values less than 4.5 or greater than 15.5.
The script for simulating the secretary interviews (Listing 2) takes on the randomly arriving candidates one by one. Following the 37 percent rule, it breaks down the @data
array into two parts. The first part, stored in @look
, contains the first 37 percent of the random numbers, which are merely for analysis of the labor market without any intention to employ; the second part (@act
) contains the other 63 percent; the employer will immediately make a decision here if one of the candidates is better than all previous encountered ones. Line 41 stops the script when this occurs.
Listing 2
secretary
If no better candidate shows up by the end of the array, the boss has lost out and has to employ the last candidate as a final option, which is what line 46 does. If the script is called with the --verbose
option, line 9 of Listing 2 switches Log4perl's logging function to debug mode to produce diagnostic messages on how the hiring process is proceeding.
The script then outputs a number of messages to standard output at run time. Figure 3 shows that the bar for the candidates during the screening stage was at 11.05, and then at 11.52. In employment mode, a number of candidates with suitability values below the previous record of 11.52 then follow, before one with a value of 11.68 gets the job.
Although the procedure has been proven to be optimal, it still often goes wrong. Figure 4 shows a run in which a candidate with an extremely high suitability value (12.84) interviews during the test phase, a value that puts all the others later in the employment round to shame. Bad luck, the boss has to take the last candidate with a rating of 9.36.
How often do these embarrassing and significant suboptimal outcomes occur in the algorithm? To measure this, Listing 3 calls the secretary
script 100 times and measures how often a candidate is given preference although they are below average. The Stats::Histogram CPAN module shows the distribution.
Listing 3
secretarystats
Figure 5 shows that the procedure finds a useful candidate in about 80 percent of the cases, including some superstars with ratings of 12 and more. However, it failed in 20 percent of cases where the boss had to take on the last candidate, whether they were a star or a clown. All told, the strategy is optimal; check out [1] for the mathematical proof.
Buy this article as PDF
(incl. VAT)
Buy Linux Magazine
Subscribe to our Linux Newsletters
Find Linux and Open Source Jobs
Subscribe to our ADMIN Newsletters
Support Our Work
Linux Magazine content is made possible with support from readers like you. Please consider contributing when you’ve found an article to be beneficial.
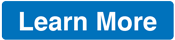
News
-
Linux Kernel 6.13 Offers Improvements for AMD/Apple Users
The latest Linux kernel is now available, and it includes plenty of improvements, especially for those who use AMD or Apple-based systems.
-
Gnome 48 Debuts New Audio Player
To date, the audio player found within the Gnome desktop has been meh at best, but with the upcoming release that all changes.
-
Plasma 6.3 Ready for Public Beta Testing
Plasma 6.3 will ship with KDE Gear 24.12.1 and KDE Frameworks 6.10, along with some new and exciting features.
-
Budgie 10.10 Scheduled for Q1 2025 with a Surprising Desktop Update
If Budgie is your desktop environment of choice, 2025 is going to be a great year for you.
-
Firefox 134 Offers Improvements for Linux Version
Fans of Linux and Firefox rejoice, as there's a new version available that includes some handy updates.
-
Serpent OS Arrives with a New Alpha Release
After months of silence, Ikey Doherty has released a new alpha for his Serpent OS.
-
HashiCorp Cofounder Unveils Ghostty, a Linux Terminal App
Ghostty is a new Linux terminal app that's fast, feature-rich, and offers a platform-native GUI while remaining cross-platform.
-
Fedora Asahi Remix 41 Available for Apple Silicon
If you have an Apple Silicon Mac and you're hoping to install Fedora, you're in luck because the latest release supports the M1 and M2 chips.
-
Systemd Fixes Bug While Facing New Challenger in GNU Shepherd
The systemd developers have fixed a really nasty bug amid the release of the new GNU Shepherd init system.
-
AlmaLinux 10.0 Beta Released
The AlmaLinux OS Foundation has announced the availability of AlmaLinux 10.0 Beta ("Purple Lion") for all supported devices with significant changes.