Backdoors in Machine Learning Models
Miseducation
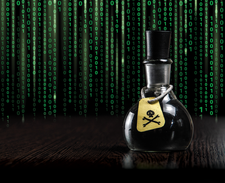
Machine learning can be maliciously manipulated – we'll show you how.
Interest in machine learning has grown incredibly quickly over the past 20 years due to major advances in speech recognition and automatic text translation. Recent developments (such as generating text and images, as well as solving mathematical problems) have shown the potential of learning systems. Because of these advances, machine learning is also increasingly used in safety-critical applications. In autonomous driving, for example, or in access systems that evaluate biometric characteristics. Machine learning is never error-free, however, and wrong decisions can sometimes lead to life-threatening situations. The limitations of machine learning are very well known and are usually taken into account when developing and integrating machine learning models. For a long time, however, less attention has been paid to what happens when someone tries to manipulate the model intentionally.
Adversarial Examples
Experts have raised the alarm about the possibility of adversarial examples [1] – specifically manipulated images that can fool even state-of-the-art image recognition systems (Figure 1). In the most dangerous case, people cannot even perceive a difference between the adversarial example and the original image from which it was computed. The model correctly identifies the original, but it fails to correctly classify the adversial example. Even the category in which you want the adversial example to be erroneously classified can be predetermined. Developments [2] in adversarial examples have shown that you can also manipulate the texture of objects in our reality such that a model misclassifies the manipulated objects – even when viewed from different directions and distances.
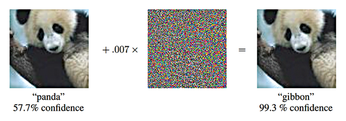
Data Poisoning Attacks
The box entitled "Other Machine Learning Attacks" describes some common attack scenarios. Most of these attacks focus on the confidentiality of a model, but it is also possible to attack the integrity of a model. In other words, you could design an attack that could influence the behavior of the model. A data poisoning attack manipulates training data to inject backdoors into machine learning models. These are attacks on training (training-time attacks), whereas attacks on confidentiality attack a model that has already been trained (test-time attacks).
Other Machine Learning Attacks
Over time, other vulnerabilities in machine learning models have become apparent. For example, it has been proven possible to extract the parameters of a model. These attacks are also referred to as model extraction attacks. The parameters encode the knowledge that the model has learned during training based on examples. Of course, training can be time consuming, especially for very large models consisting of hundreds of millions or even billions of parameters, which can be very expensive. For example, it is estimated that the research and development of GPT-3, a transformer model with 175 billion parameters, cost $10-20 million.
Expensive models like this are only rarely freely available to the public. Instead, they are often operated in the cloud, in a protected infrastructure, as machine-learning-as-a-service, so that no one can access the parameters. If you want to use the model, you are given access to its API for a charge. However, if certain conditions are met, the parameters can be extracted from a model by clever use of the API. Once you have the parameters of a model, there is no longer any reason to pay for the cloud service because you are able to run the model yourself.
A membership inference attack is an attack that can extract information from a model that is confidential and should not be public. A clever query can find out whether certain data was used to train a model – for example, data related to a specific person. Suppose a model has learned to recognize a disease based on patient data, and the model was trained with the data of people who are suffering from this disease. If you can determine that a particular person is in the data set, you know that person has that disease.
Model inversion attacks are attacks that reconstruct the data used for training from a model [4]. To create a model that can recognize faces, the training data set needs to contain sample photos of each person you want the model to recognize. Figure 2 (left) shows photos that could occur in such a data set. Given access to the parameters of the model trained with these photos, you can reconstruct a recognizable image of each person included in the data set (Figure 2, right).
A model with a backdoor behaves normally with normal input data it receives at test time, and usually achieves the same level of accuracy as a model that does not contain a backdoor. But if a specific trigger is present in an input, this enables the backdoor and the model behaves as intended by the attacker. A model then recognizes a dog instead of a cat, for example.
For such an attack, a hacker needs access to the training data. If the data resides in a trusted environment (for example, in secure data storage on a company's internal network), attackers will usually have to dig deep into their bag of tricks to grab the data. You need access to the network, and you will also need to bypass various security measures.
Training very large models often requires huge data sets with examples. The data usually needs to be labeled up front. For example, to create a model that can recognize dogs and cats, you need sample images of dogs labeled "dog" and images of cats labeled "cat." However, labeling very large data sets is very time consuming. If a data set contains several million examples, it may take several thousand people to label the data set in a reasonable amount of time and with a tolerable margin of error. Many universities and companies cannot do this just relying on their own staff and often resort to crowdsourcing. One example of a crowdsourcing platform is Amazon Mechanical Turk [5].
Large tasks are broken down into many small subtasks. A crowdsourcing platform then provides these subtasks. Any person can select and edit a subtask on the Internet (for example, labeling images). After the work is done, the volunteers are credited for their services. Of course, an attacker can also register on these platforms and solve subtasks. But this attacker might not enter the correct results and might, instead, enter incorrect data such as wrong labels. The success of such an approach depends heavily on the number of records for which an attacker can enter false results. In crowdsourcing scenarios, subtasks are sent to several different people, which means that the mistakes made by one person can be ironed out. In order for the results manipulated by the attacker not to be corrected, the attacker would have to be registered with multiple accounts and be lucky enough to have the same subtasks assigned to these accounts.
Another option for attackers would be to manipulate existing data and publish the manipulated data on the Internet. Large volumes of data for training come from the Internet. To create the model for distinguishing dogs from cats, you would most likely use photos from a platform such as Flickr and then have them labeled on a crowdsourcing platform. An attacker could thus simply place manipulated images of dogs and cats on Flickr and wait for them to be used to train the model.
Badnets
Suppose the attacker has found a way to manipulate training data. The following simple example shows how the manipulated data can be used to inject a backdoor into a Convolutional Neural Network (CNN) that recognizes handwritten digits. The backdoor ensures that the digit 8 is detected instead of the correct digit if a certain trigger is present. The example is based on the paper "Badnets: Identifying Vulnerabilities in the Machine Learning Model Supply Chain," by Tianyu Gu, Brendan Dolan-Gavitt, and Siddharth Garg, which was published in 2017 [6].
Consider the possibilities of this attack. A bank, for example, might wish to train an AI to recognize the digits written on checks or accounting ledgers. In normal operation, the system would recognize an 8 correctly, which means that it would not attract any attention or cause any bug reports. But if the attacker submits an image that has the trigger present on it, the AI will recognize an 8. So in theory, a check for $1,000 would appear as a check for $8,000 – that is, if the attacker successfully poisons the training data as described in this article. Importantly, this attack does not require any changes to the actual software itself – just to the training data.
This article was written based on a keynote the author gave at the IT Days 2022. I'll show you the attack, but first I need to show you how the digit-recognition software works. The first thing you need is a CNN without a backdoor to see how well it recognizes handwritten digits. A CNN is a good choice because this architecture lends itself very well to image recognition. Then you need to train the network using examples of handwritten digits with MNIST [7], a freely available data set used for training handwriting recognition on machine learning frameworks. The data set contains a total of 70,000 examples of handwritten digits. Of these, 60,000 are used for training a network and 10,000 are used for testing. Each example is a simple grayscale image with 28x28 pixels. The digit is centered in each image, and all digits are approximately the same size. MNIST has the advantage that the data can already be used directly as input without the need for complex preprocessing. Figure 3 shows some examples.
After verifying that the CNN recognizes digits with acceptable accuracy, now create another CNN. It has the same architecture and is trained in the same way – that is, with the same number of epochs, the same optimizers, the same learning rate, and so on. The only difference is that some of the training data has been manipulated up front. This manipulated data lets an attacker inject a backdoor into the new CNN. The goal: The new CNN needs to achieve a similar accuracy to the first CNN, which does not contain a backdoor. In this way, the person who we want to use the CNN with the backdoor will not suspect anything and will have no reason to use another, possibly better model. Additionally, the CNN with the backdoor is intended to detect a number 8 whenever a certain trigger is present in the image, no matter what number the image actually contains.
Buy this article as PDF
(incl. VAT)
Buy Linux Magazine
Subscribe to our Linux Newsletters
Find Linux and Open Source Jobs
Subscribe to our ADMIN Newsletters
Support Our Work
Linux Magazine content is made possible with support from readers like you. Please consider contributing when you’ve found an article to be beneficial.
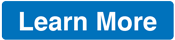
News
-
Red Hat Adds New Deployment Option for Enterprise Linux Platforms
Red Hat has re-imagined enterprise Linux for an AI future with Image Mode.
-
OSJH and LPI Release 2024 Open Source Pros Job Survey Results
See what open source professionals look for in a new role.
-
Proton 9.0-1 Released to Improve Gaming with Steam
The latest release of Proton 9 adds several improvements and fixes an issue that has been problematic for Linux users.
-
So Long Neofetch and Thanks for the Info
Today is a day that every Linux user who enjoys bragging about their system(s) will mourn, as Neofetch has come to an end.
-
Ubuntu 24.04 Comes with a “Flaw"
If you're thinking you might want to upgrade from your current Ubuntu release to the latest, there's something you might want to consider before doing so.
-
Canonical Releases Ubuntu 24.04
After a brief pause because of the XZ vulnerability, Ubuntu 24.04 is now available for install.
-
Linux Servers Targeted by Akira Ransomware
A group of bad actors who have already extorted $42 million have their sights set on the Linux platform.
-
TUXEDO Computers Unveils Linux Laptop Featuring AMD Ryzen CPU
This latest release is the first laptop to include the new CPU from Ryzen and Linux preinstalled.
-
XZ Gets the All-Clear
The back door xz vulnerability has been officially reverted for Fedora 40 and versions 38 and 39 were never affected.
-
Canonical Collaborates with Qualcomm on New Venture
This new joint effort is geared toward bringing Ubuntu and Ubuntu Core to Qualcomm-powered devices.